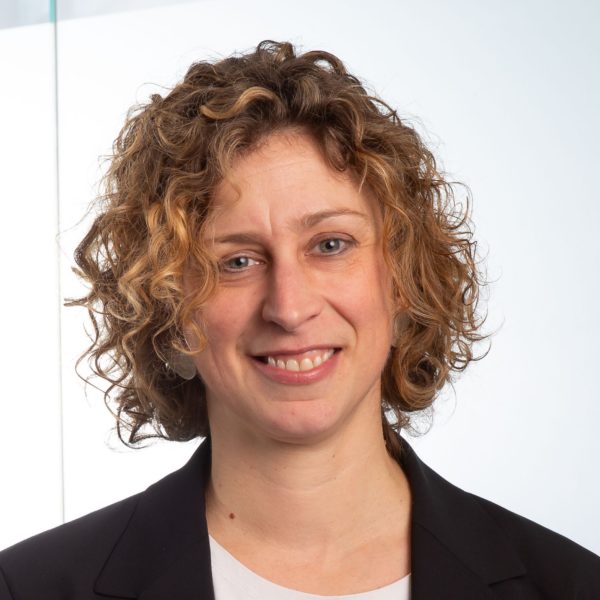
Sara Gosline. (Photography by Scott Eklund/Red Box Pictures)
What is your name, title and institutional affiliation?
Sara Gosline, Ph.D.
Computational Biologist, Pacific Northwest National Laboratory
What is your role, ie what do you do?
I work with scientists, physicians, engineers, and chemists to interpret the results of high throughput assays on biological samples. I work in teams that apply existing computational tools or develop new ones to address specific needs of the dataset, such as technical bias or low sample size.
In NF, I use my technical skills to generate, normalize, curate, and analyze pre-clinical data to fuel drug discovery – either by analyzing existing data or helping scientists develop new model systems such as patient derived xenografts for MPNSTs, organoid models for cNFs, or even microtissue models. The question I’m most commonly asked is ‘How can this data help us get to a new drug?’ The answer is always different.
Please share your favorite discovery from your NF research. What makes this particular discovery rise to the top?
I only moved into NF research ~5 years ago, and mostly on the back end. But I think my favorite finding is the confirmation of the low mutational burden of cNFs in the Scientific Data manuscript. In the field of targeted oncology, mutations are a prime target of drugs, and the lack of recurrent mutations stymied the field. However, tumors don’t grow in a vacuum and require a supportive environment to provide nutrients and growth signals. Confirming this absence has justified further exploration of the microenvironment via tumor deconvolution (Banerjee et al.) and single cell RNA-seq (Pluquet’s paper). This finding also fueled my own interests in the construction of better models of NF1-related disease to better account for the microenvironment.
In your mind, why is data sharing/open science important? How has this practice improved your research?
Data sharing, particularly in rare disease, helps the science move forward faster. In my own research I’ve seen how standardizing the RNA-seq data across NF samples on the NF Data Portal through a single pipeline has made it possible for us to integrate these data in computational models (Banerjee et al.), MPNST model studies (Larsson et al., to be submitted) and cNF organoid data (Nguyen et al., to be submitted). As we stitch together additional datasets we can build a more concrete picture of NF molecular signals.
Where do you see NF research going in the next five years?
I think we have just scratched the surface with drug testing and our understanding of the microenvironment. I’m looking forward to building better models for drug testing and also seeing which molecular pathways are altered by the drugs in NF1-deficient systems. While I can’t develop better systems with my expertise, I hope my signaling pathway knowledge and modeling can help identify specific kinases that can be targeted by drugs, either alone or synergistically with other drugs.
Sara Gosline received a BA in computer science from Columbia University and spent two years working in the software field before returning to graduate school full-time. She received her master’s degree and PhD in computer science from McGill University, with a specialty in bioinformatics. Then, she moved to the Massachusetts Institute of Technology where she worked as a postdoctoral researcher in the Department of Biological Engineering with Ernest Fraenkel and Phillip Sharp; there, she focused on employing computational algorithms to disentangle biological data from cancer and other diseases.
Since completing her training, she worked at Sage Bionetworks, a non-profit that focuses on accelerating the pace of biomedical research through enabling data sharing and collaboration. She focused on supporting scientific discoveries in rare diseases. Gosline has since become a research scientist at PNNL where she continues her research on rare diseases, as well as working with other large molecular datasets, specifically trying to employ novel algorithms in cancer and other diseases.